Presentation of the research paper “Skeleton Image Representation for 3D Action Recognition based on Tree Structure and Reference Joints”, at Main Track.
[/trx_title]
Presentation of the research paper “Skeleton Image Representation for 3D Action Recognition based on Tree Structure and Reference Joints” by Carlos Caetano, at Main Track.
32nd Conference on Graphics, Patterns and Images SIBGRAPI 2019.
October 28th to 31st | Rio de Janeiro.
In recent years, the Computer Vision research community has investigated how to model temporal dynamics in videos for the recognition of human activities in 3D. To this end, two main approaches were researched.
(i) Recurrent Neural Networks (RNNs) with Long-Short Term Memory (LSTM).
(ii) skeleton image representations used as input for a Convolutional Neural Network (CNN).
Although RNN approaches present excellent results, such methods lack the ability to efficiently learn the spatial relations between the skeleton joints. On the other hand, the representations used to feed CNN approaches present the advantage of having the natural ability of learning structural information from 2D arrays (i.e., they learn spatial relations from the skeleton joints).
To further improve such representations, we introduce the Tree Structure Reference Joints Image (TSRJI), a novel skeleton image representation to be used as input for CNNs.
The proposed representation has the advantage of combining the use of reference joints and a tree structure skeleton. While the former incorporates different spatial relationships between the joints, the latter preserves important spatial relations by traversing a skeleton tree with a depth-first order algorithm.
Experimental results demonstrate the effectiveness of the proposed representation for 3D action recognition on two datasets, achieving state-of-the-art results on the recent NTU RGB+D~120 dataset.
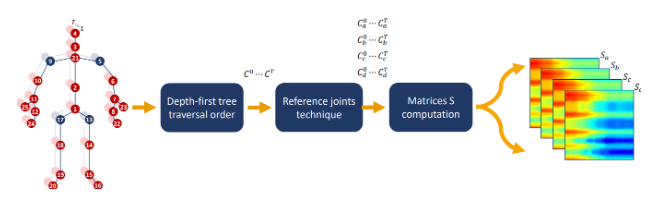
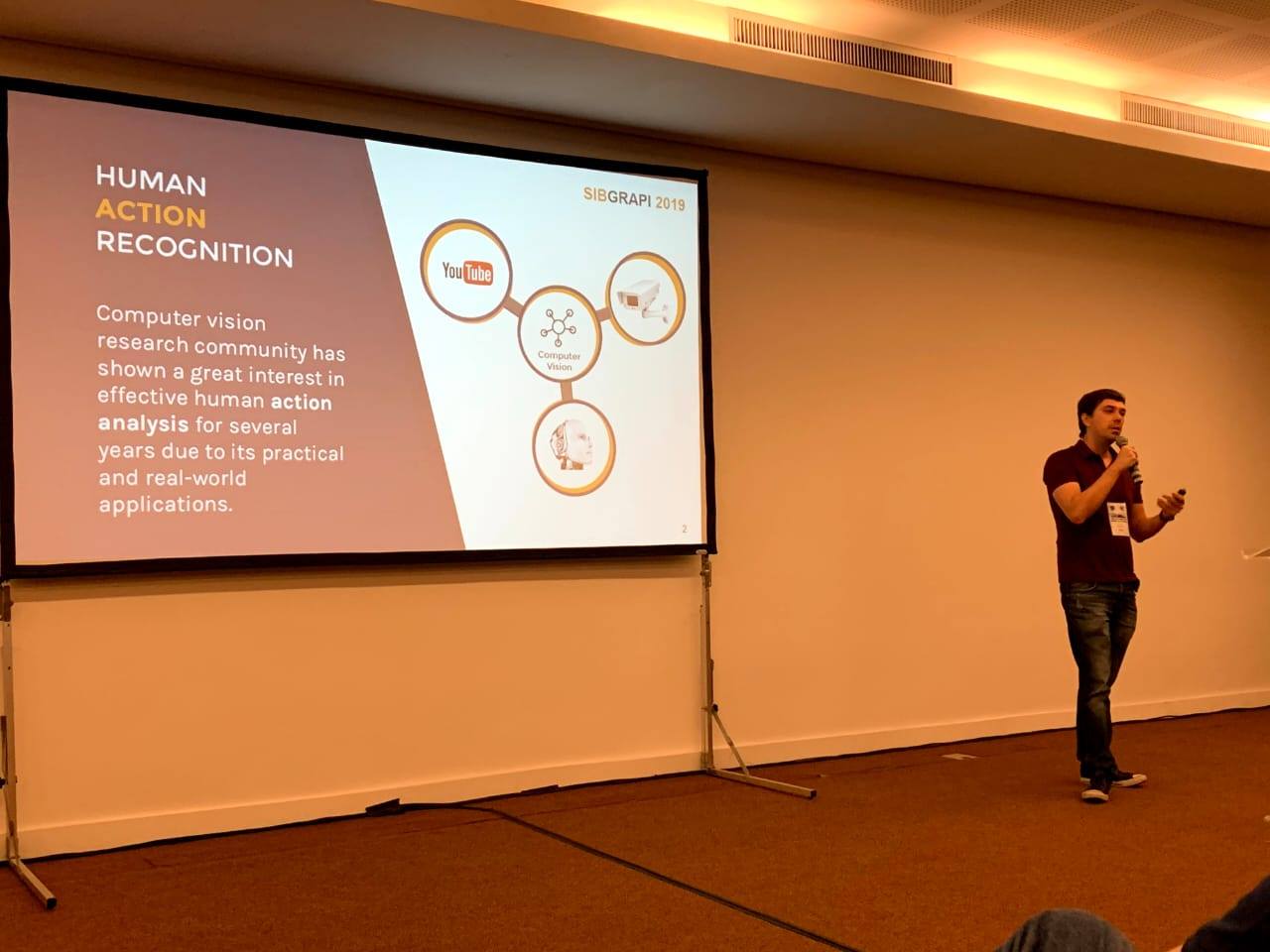